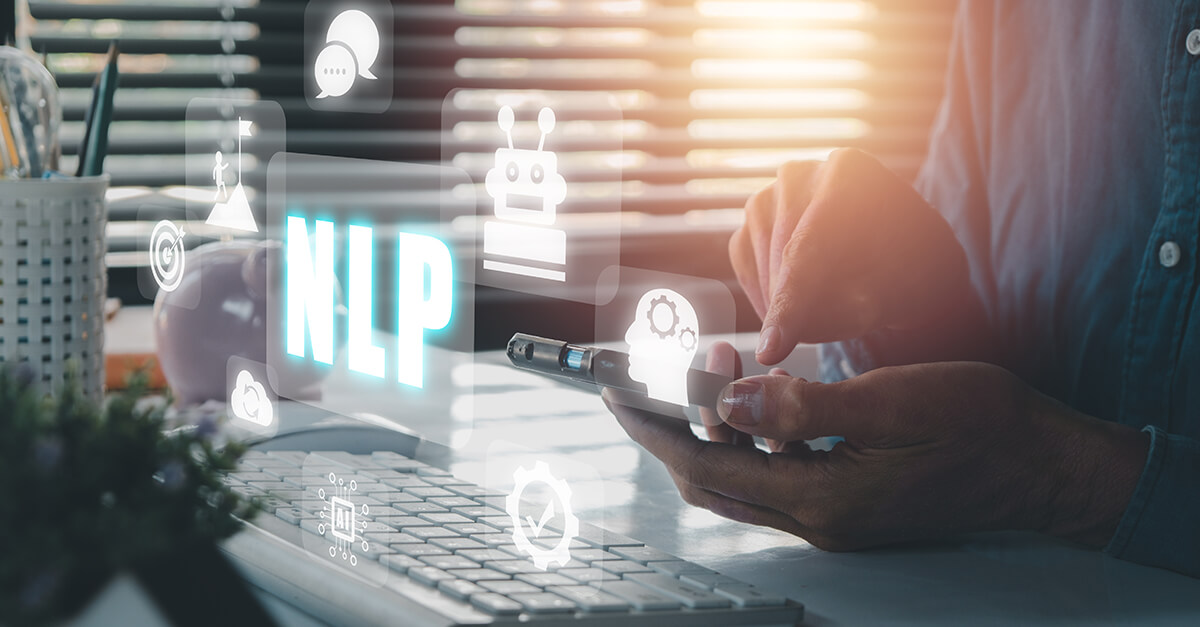
6 Steps to Making NLP + RPA a Priority
Within the domain of intelligent automation (IA), complementing automation with Natural Language Processing (NLP) should be top of mind for many reasons.
NLP extends existing RPA capabilities
Adding Natural Language Processing to an IA program is like adding another module of RPA rather than onboarding a whole new solution. Not only will it assist with structuring unstructured data required for automating downstream processes, but it will also identify at scale more processes and areas to bolster the automation pipeline (effectively acting as a process mining tool).
Firm Business Case
In our recent whitepaper about the economics of NLP + RPA, we concluded that with minimal investment in NLP, IA programs can achieve 3-year ROIs of about 200% and 12 to 24-month payback ratios of 1 and 2, respectively. These expected ROI and payback metrics are consistent with those targeted by executives in IA.
Fast Time to Value
Almost every organization has a set of unstructured data (e.g., emails, free text fields) at the ready, just waiting to be mined for insights and applied to automation. And business SMEs without experience in NLP technology, rather than data scientists, can quickly develop models and start getting value from solution.
While the benefits of NLP in conjunction with RPA are well understood, a common challenge faced by IA programs is figuring out how to onboard an NLP solution. Given our experience in the space, here are 6 steps to follow when launching an NLP solution within your IA ecosystem:
Step 1: Confirm the maturity of your program
It can be tempting to bring in an NLP solution immediately but there are two considerations to make before the ball starts rolling.
The first consideration is around the maturity of the IA program. If there are multiple automations in place, where most of the challenges of the platform itself have been shuck out, then it is still appropriate to proceed to the next step of onboarding an NLP solution. That is because it is best to avoid burdening IT with setting up multiple platforms at the same time.
The other consideration is around the maturity of the analytics program (if there is one). If the organization already has data scientists, it will be important to engage with them early on in the NLP solution onboarding discussions as it can fall within their wheelhouse. Historically, very few NLP models built in-house by data scientists have success since it is a tough challenge to fix by nature and even once a model makes it to production, it can quickly become outdated and difficult to maintain; potentially, these data science teams may embrace an NLP tool with a proven track record and provides them with greater ability to glean product, customer, revenue, and process intelligence.
Step 2: Perform an NLP opportunity scan & assessment
Once the maturity level of the IA and analytics programs are confirmed, it is helpful to perform an initial scan of opportunities in the organization that are best geared for NLP + RPA. Below we have a list of “green flags” to listen for, when performing the scan in the organization to see where NLP + RPA is most appropriate.
- Teams collectively spend lots of time triaging and extracting information from emails at scale
- Teams have to read emails AND attachments within emails
- Teams are using structured forms but there they still have issues
- There are manual referrals for current automations that require SME understanding
- The current process mining suite is not adequate and is struggling to identify where the process bottlenecks are
- The data analytics teams need more data to play with
The first green flag listed above is very important; any time there is a shared inbox like with an IT service desk or customer support center, it is a ripe candidate for both NLP and RPA.
Another key call out is with structured forms that still have issues. Often we see these forms that the most important information is contained in a catch-all description field (e.g., problem description, general comments) and having an NLP tool that can structure the info in that field is critical for downstream processing.
If any of these green flags registered for a given team or process within the organization, an assessment consistent with process assessments performed by an IA business analysts should be performed. In particular, info around average monthly case volumes (i.e., number of unstructured communications) and handling times (i.e., time it takes to process a case) should be obtained for each process. Typically, we would like for at least 10 full time equivalents (FTEs) for a team/process with unstructured data before proceeding to the next step.
Step 3: Conduct an NLP learning session and demo
Once we see the potential value of NLP in a few different teams and processes, business and technology stakeholders will now need to be educated in what NLP is, what are some solutions out there, and what is the value from NLP. Just like with RPA, in order for NLP solutions to be successful, there will need to be stakeholder buy-in at all levels and teams involved.
At Reveal Group, we have spent a great deal of time educating clients on these solutions and would be more than happy to conduct NLP learning sessions and demos upon request.
Below are some handy video links where we discuss some specific NLP tools and provide corresponding demonstrations.
Tech Talks
— Tech Talks: RPA & NLP with Re:infer – Reveal Group
— Tech Talks: Structuring Unstructured Data for Automation featuring Expert.ai – Reveal Group
Demo Material for Re:infer and Expert AI
— Expert.ai Demo – Reveal Group on Vimeo
— Re:infer Demo – Automated Flight Delay Compensation (vimeo.com)
Step 4: Select a vendor based on NLP requirements
Before selecting a vendor, it is important to not only narrow down opportunities for a proof of technology, but as well as outlining infrastructure and information requirements for the NLP Tools. As there is no “one-size-fits-all” NLP tool out there, the agreed upon requirements will greatly influence which tool is selected. For example, some tools require GPUs while other tools do not; some have built-in analytics and OCR capabilities.
It will also be important to collect these requirements not just from the IA leads, but also from the business, IT, and data scientist stakeholders.
Given our experience evaluating several NLP tools in the space, we can provide the selection team with dimensions to best assess NLP tools, allowing for prioritization of specific dimensions given specific use case and platform needs.
Step 5: Deliver an NLP +RPA solution
Once an NLP is selected, building the NLP model and integrating it with RPA solution is the next step. It is generally helpful to pick a simple use case (e.g., email triage) and ideally one that already has an RPA solution in place but has a manual step due to one of the inputs being unstructured currently. Fortunately, NLP models have a much shorter development time than RPA (average development time for an NLP model is 4-6 weeks, compared to that of RPA being 6-10 weeks) and can be done concurrently with RPA delivery as well.
Step 6: Showcase the solution
Just like RPA, being able to showcase the NLP+RPA solution to other business areas will be helpful to expand your intelligent automation program even further. Communicating its business value in terms of reduction in manual effort, response times, and errors will still be important; however, even more so will be showing the solution in real time where the bot sends the unstructured communication through the NLP model, gets back labels and data items, and uses that info for downstream processing.
If you have any questions on how to make NLP+RPA a priority in your organization or would like a demo of NLP solutions, let’s chat!